Radiologist Pouneh Razavi looks for any blips in the X-ray image that might be signs of breast cancer when she reads a patient’s mammography. After that, a second reader examines the picture, and the two compare the outcomes.
However, the second reader is artificial intelligence rather than a real doctor. AI software has been Razavi and her colleagues at the Johns Hopkins School of Medicine’s second pair of eyes since March.
Since the software is still in its infancy, both her team and it may learn from one other. Pictures from Razavi’s work might be used to teach the AI on errors that it overlooked, allowing it to get better over time. Although Razavi’s colleagues are currently gathering data, the verdict is yet out on its effectiveness, she says, “we’re excited about it.” And so are her patients. “So far, it’s just fascinating everyone,” says Nancy Shute, Editor in Chief of Science News, who had her first AI-analyzed mammography from Razavi in May.
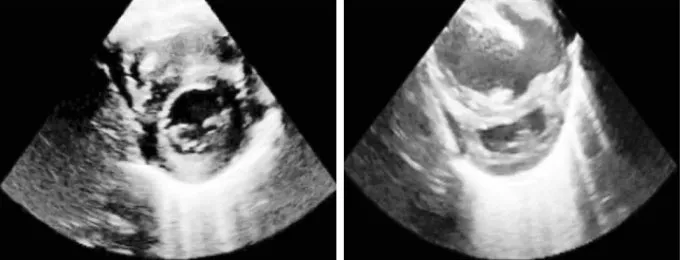
AI is being used to support medical imaging beyond mammograms. Physicians are scanning chest X-rays, ultrasound recordings of newborns’ hearts, and other medical images with this technique. “Imaging is leading the way,” according to Stanford University radiologist Curtis Langlotz, who spoke at The New Wave of AI in Healthcare symposium in New York City in May. AI technology in medicine is expanding quickly.
About 75% of the almost 900 AI-related medical gadgets that the US Food and Drug Administration has authorized since 1995 have been tied to radiography. However, Mert Sabuncu, a Cornell University technician, pointed out during the meeting—which was organized by the New York Academy of Sciences and the Icahn School of Medicine at Mount Sinai—that not every clinic in the nation is connected to the technology. “I would say we’re just starting to deploy them,” he actually stated.
ascending
The quantity of AI-related medical gadgets that the US approves each year. Particularly in recent years, the Food and Drug Administration has experienced significant growth. The FDA had authorized 691 of these devices as of October 2023 (and roughly 200 subsequently). In order to screen pap smears, a regular test to check for symptoms of cervical cancer, the first device was approved in 1995.
Sabuncu and other academics discussed the possible benefits and drawbacks of artificial intelligence (AI) in medical imaging with Science News. This is what we discovered.
Medical image data is abundant, making AI a great fit.
When Langlotz gets to the hospital early on a Saturday morning, he usually finds 150 patient photos ready for his inspection. Examining chest X-rays, he will search for fluid in the lungs, pneumonia, atypical nodules, and cracked ribs.
It’s a task requiring training, concentration, and attention to detail, but even professionals can make blunders. According to studies, a radiologist’s daily error rate could be between 3 and 5%. According to research published in the European Journal of Radiology in 2023, overwork is typically the cause of these mistakes. “Help is needed,” Langlotz declared.
It’s unlikely that the issue will get better very soon. As more patients have scans, more images are being obtained by doctors than in the past, and each image contains an increasing number of pixels. According to Sabuncu, radiologists find that the needle in a haystack analogy is not very helpful when looking for a suspicious region on an X-ray. More accurately, it’s like “looking for a needle in a haystack when you really don’t want to miss anything and you’re under a lot of time pressure and the patient’s health is at stake.”
An enormous challenge for humans could present a golden opportunity for AI. Scientists can teach artificial intelligence (AI) computer models to look for particular elements in a person’s scan, such as a smudge in a breast image or indications of lung disease on an X-ray, by using vast amounts of high-quality digital photos.
These models can enhance the precision and efficacy of radiologists. They can also identify images that appear particularly concerning, enabling medical professionals to prioritize patients according to which ones require urgent care. For example, Langlotz said, “I’d love to know which of those is more likely to have a problem,” when he saw his cases piled up in the morning.
AI could assist medical professionals in identifying illness symptoms.
A radiologist performing a chest X-ray can be looking for indications of an infection or lung cancer. However, information concerning other facets of the subject’s health is concealed in plain sight in that picture. AI algorithms could be able to extract more data from each scan. Radiologists aren’t mining the richness that exists in image data, according to Sabuncu. “A lot of information is being left on the table.”
Consider heart illness. Typically, medical professionals use factors like blood pressure and cholesterol levels to determine an individual’s risk. However, without the missing data, those computations are not possible. AI may be able to help with that by extracting cardiac data from standard X-rays.
“As radiologists, we’ll be extracting information from images that we typically don’t extract.”
Curtis Langlotz
Researchers reported in the April Annals of Internal Medicine that an AI model analyzed chest X-rays from over 8,900 adults ages 50 to 75 and identified who could subsequently develop a heart attack or stroke using a set of previously gathered pictures and follow-up data. This exemplifies “opportunistic imaging,” in which artificial intelligence searches images for medical information other than the initial X-ray goal. Compared to other individuals reviewed, the 4,200 or so people flagged by the AI had a 1.5-fold higher chance of developing a major cardiovascular issue during the next ten years.
A different X-ray scanning AI was utilized in a related study in 2023 to effectively identify type 2 diabetes. In that instance, the AI was trained on hundreds of thousands of chest X-rays, and researchers identified which images belonged to patients who had the illness. The AI was able to identify the same distribution of fat in those individuals, particularly around the liver and heart. It’s not something that medical professionals usually check for during a standard X-ray. But according to Langlotz, that’s the main idea of AI in imaging. “As radiologists, we’ll be extracting information from images that we typically don’t extract.”
The authors of the study note that an AI tool like this could benefit those who don’t have access to conventional medical treatment. One day, these individuals may get a normal chest X-ray during an ER visit, which could reveal problems other than the one that initially brought them to the hospital.
According to Sabuncu, AI has yet another application: it can enable us to look more closely at the past in order to peek into the future. Today, the majority of AI image analyses make a diagnosis based just on one image, often without taking into account a patient’s prior scans. Artificial intelligence systems that analyze the evolution of a patient’s medical image are of interest to Sabuncu. “This entire clinical history must be taken into consideration,” he stated.
For example, his team recently developed a program that searches through an individual’s mammograms over time. The model was assisted in identifying worrisome lesions by these historical data. In a paper submitted on April 29 to arXiv.org, the team found that the AI was more than 80% accurate in identifying individuals who would develop cancer within the next five years from those who wouldn’t. The findings will be presented in October at the Medical Image Computing and Computer Assisted Intervention conference in Marrakesh, Morocco. With patient outcome data that the AI was not privy to, the researchers evaluated the AI’s performance.
According to Julia Vogt, a computer scientist at ETH Zurich, AI can also assist scientists in sorting through even more intricate visual data, such as ultrasound films of newborn babies’ hearts. Although it’s challenging and time-consuming, skilled clinicians may identify infants who may have cardiac issues by watching such films, she says. German clinic doctors asked her team if they might be of any assistance.
An AI model developed by Vogt’s team examines ultrasound images of newborns in addition to other data. Her team reported on February 6 in the International Journal of Computer Vision that the model could correctly identify which newborns had a cardiac condition called pulmonary hypertension about 80% of the time in a test that used videos from 192 babies who had previously been examined by pediatric cardiologists. Such problems may often be remedied with supplemental oxygen or drugs, and Vogt adds, “we can actually make a huge impact on newborns” by recognizing the issue early.
Although AI might benefit patients, doctors won’t be replaced by it anytime soon.
It’s not a simple task to introduce new technology into the healthcare industry. It doesn’t mean the technology is ready to use, according to Sabuncu, even though researchers may publish studies of AI models that can recognize cancerous cells in brain cancer samples or parasitic worm eggs in children’s feces. “There is still a ton of work to be done.”
AI models must be verified and examined in environments different from those in which they were trained. To use them, one must possess both clinical and technical expertise. To get approved, they must go through the regulatory procedure. And ideally, according to Sabuncu, they would be used in the actual world and their effectiveness assessed.
The model that Vogt’s team created isn’t ready for a broad release, just like the majority of AI systems that are currently documented in scholarly publications. It must first be trained on more data by the researchers. There are now too many factors, such as the kinds of ultrasound machines used and the ways in which various institutions record footage, that could cause confusion. According to her estimation, it might take three to five years to validate the model through additional training and trials.
However, Vogt claims that’s what’s required to expand the model’s use in hospitals. “You need evidence to show whether it is truly helpful.”
According to Sabuncu, enthusiasm around AI can arise from public expectations about technological advancements. “AI has a lot of potential, there is no doubt about it,” he stated. He believes that technology will one day have a significant influence on medicine. “I don’t think we’ll see that impact very rapidly,” he added.
According to Vogt, intelligent and cautious application of AI technologies may eventually result in more effective health care. She acknowledges that artificial intelligence (AI) is not magic, but it can help with specific issues. AI might, for instance, allow doctors to spend more time with their patients by reducing workloads. She remarks, “That would be a huge achievement.”
Though it’s a worry they’ve all heard, neither Vogt nor the other experts Science News spoke with see AI replacing doctors anytime soon. Some patients believe that after receiving a scan, they will solely communicate with an AI; humans won’t be involved in any way, according to Razavi. They mistakenly believe it to be similar to Whole Foods’ self-checkout station, but that is untrue, she claims. Breast imaging radiologists read every image at the Johns Hopkins imaging centers.
Her team is hopeful that AI can decrease false positives, prioritize medical tasks for physicians, and enable early cancer detection in patients. The potential benefits of AI will vary depending on its level of development, how clinics use it, and who has access to it. According to Razavi, some breast imaging facilities charge patients an additional $40 or more to have AI examine their results. Free of charge, Johns Hopkins does it.
“We don’t want to deny that to someone if there is a technology that can help find a small cancer,” says Razavi.